Neural network patentability before the European Patent Office
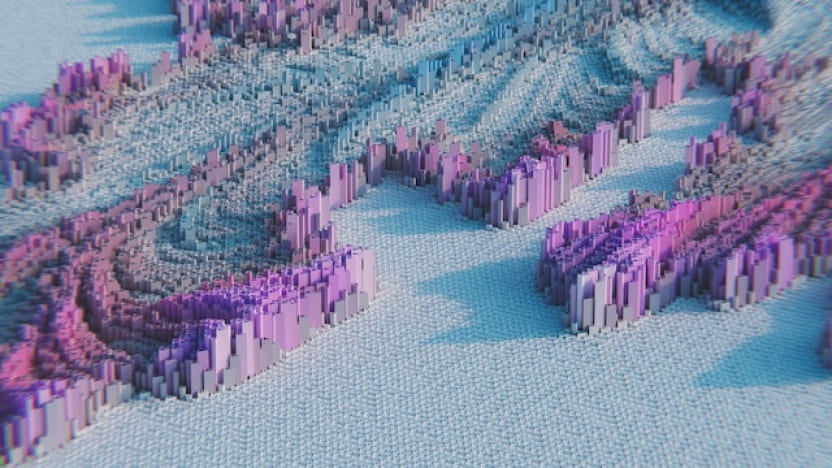
A recent decision by the European Patent Office on neural network patentability has interesting implications for artificial intelligence and other computer-derived innovations, says Martin Kohrs.
Decision T 0702/20 by the Board of Appeal of the European Patent Office (EPO) is interesting for several reasons. Firstly, because it concerns neural network patentability, the basis of many artificial intelligence (AI) applications and, therefore, a very topical issue. Secondly, because it comes after the decision G1/19 by the EPO’s Enlarged Board of Appeal, which concerned the patentability of computer-implemented simulations based on mathematical models.
The case considers:
- the nature and technical character of a neural network in general; and
- more specifically, the technical effect of differences with the state of the art in the functioning of a computer used for implementation.
The invention and the applicant's arguments
The invention which was the subject of the appeal in T 0702/20 concerns a hierarchical neural network device. This network is characterised in particular by layers of neurons which are only partially coupled ('loose coupling') before the training phase of the network. The partial coupling is also based on a sparse parity-check matrix. These are the two main features differentiating the claim from the prior art.
According to the claim, the invention enables, among other things, a limiting of the computational resources required during the training phase of the network to prevent a phenomenon known as 'overlearning'. Overlearning occurs when an oversized neural network absorbs the training data at too detailed a level and has difficulty in generalising afterwards.
The applicant argued, among other things, that:
- Machine learning has, in general, "a technical purpose" and solves "a well-defined technical problem using mathematical means". An analogy with the field of cryptography is put forward; indeed, cryptographic processes are not considered to be abstract, even though they are often based primarily on mathematical algorithms, and even though the input and output data of the process are abstract.
- The claim relates to a computer, and so a specific technical implementation is present. The new features increase the learning capabilities and efficiency of the device (smaller storage capacities are sufficient) and these are technical effects. An implementation on less efficient devices becomes possible. Decision G 1/19 states in particular that better use of storage in a computer can be a technical effect.
The decision of the Board of Appeal
The Board of Appeal did not agree with the applicant on any of the arguments put forward.
- The Board of Appeal first recalled the nature and structure of a neural network, which consists of layers of neurons, each of which receives input data and produces output data. According to the Board, the structure of the network determines the class of mathematical functions that the network can represent. As such, the neural network is not considered to have a technical character, therefore..
- Furthermore, the analogy of machine learning and cryptography was not considered valid. Indeed, machine learning is not linked to an implicit technical use, unlike cryptography, which implies increased security in the transmission of encrypted data.
- The Board was of the opinion that there is no 'implied further technical use' of the output data, therefore, as might have been the case for some of the examples of simulations based on mathematical models described in G1/19.
- Although the claim does not relate to an object excluded as such from patentability (in this case a computer), the claimed implementation does not, according to the Board, require any adaptation of that computer. No particular hardware structure is claimed, or even described.
- Moreover, a reduction in the storage capacity required would not in itself have any technical effect: a network comprising, for example, a single neuron would require few resources, but could not learn complex data either.
- As for the supposed advantage of overlearning, the Board stated that it is not described how this is achieved. Overlearning generally depends on the characteristics of the training data and these characteristics are not mentioned in the context of determining the partial coupling.
What can we conclude from the Board’s decision?
The Board of Appeal has strictly applied the existing rules and rejected the appeal. It did not consider the new 'structure' of a neural network sufficient to be considered technical. In the Board’s view, it is a pure mathematical algorithm.
However, the Board did not rule out that an invention based on a neural network could solve a technical problem. It pointed out that, in most cases, this would require the network to be sufficiently specified, in particular with regard to the training data and the technical tasks it is intended to perform.
For further information or specific advice on neural network patentability, please speak to your Novagraaf attorney or contact us.
Martin Kohrs is a French & European Patent Attorney based at Novagraaf in Paris.